We look forward to MLonMB on November 10, 2021 from 9am-3:30pm at the UCSC Hay Barn (coffee and fruit will be available from 8am-9am). Our last requests of you prior to the meeting are described below. They include input on your dietary requirements, parking, and a bit more.
Logistical info
This Google doc describes logistics like parking and COVID protocol. We will supplement it when asked.
Please fill out this form to provide us with your dietary information and other salient info. We request this information by October 20th.
Introductory Slide
We are asking each participant to provide a single slide introducing themselves. At a minimum, please provide a photo, a brief bio, and a few interesting tidbits related to your attendance at the meeting.
Here is an example from one of the organizers.
You can copy that Google Slide and then edit. Or generate your own PDF with any software. We will generate and share the slide deck for the full set of attendees.
We request this slide by November 1st. Send it to cjhangen@ucsc.edu with subject “MLonMB slide”.
Agenda
A draft of the agenda is below. It emphasizes interaction, i.e. meeting each other and holding informal discussions.
Talks
Time
|
Activity
|
Participants/Notes
|
8-9
|
Coffee/fruit
|
All
|
9-9:05am
|
Welcome (Refer to attendee intro slide packet, Intro to Idea Boards)
|
All
|
9:05a-9:30a
|
Introductory Bingo
|
All
|
9:30a-10:30a
|
Data Talks (10 x 6 minutes)
— Who I am and represent
— Data I have
— Why I obtain it
— What I aim to do with it
|
Capture on Idea boards
|
10:30-10:48
|
Break
|
|
10:48-12pm
|
ML Talks (12 x 6 minutes)
— Who I am and represent
— Technical expertise
— What it is useful for
— Data I’d love to work on
|
All
|
12-1p
|
Lunch
Review Idea Boards
|
All
Organizers
|
1-2pm
|
Results from idea boards, break into smaller groups
|
Capture on idea boards focused on “areas of interest”
|
2-3pm
|
Idea group discussion / walk-around?
|
|
3:15pm-3:30pm
|
Wrap-Up + Future engagement
|
Avoid Friday!
|
3:30pm-end
|
Collaboration building
Hike (for those that want)
Otherwise at site
|
|
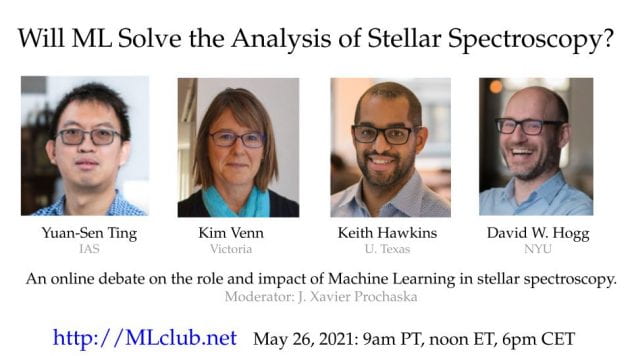
When: 9am PT, noon ET, 6pm CET
How: http://MLclub.net
Who:
- Yuan-Sen Ting (IAS)
- Kim Venn (Victoria)
- Keith Hawkins (Texas)
- David W. Hogg (NYU)
Title: “Will Machine Learning Solve the Analysis of Stellar Spectroscopy?”
Abstract: An online debate on the benefits and downsides that Machine Learning can play in stellar spectroscopy.
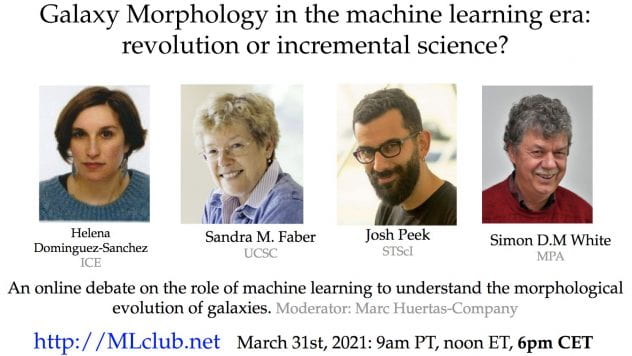
When: 9am PT, noon ET, 6pm CET
How: http://MLclub.net
Who:
- Helena Dominguez-Sanchez (ICE)
- Sandra M. Faber (UCSC)
- Josh Peek (STScI)
- Simon D.M. White (MPA)
Title: “Galaxy Morphology in the machine learning era: revolution or incremental science?”
Abstract: An online debate on the challenges of photometric redshift estimation and the role Machine Learning can play in wide-field surveys.
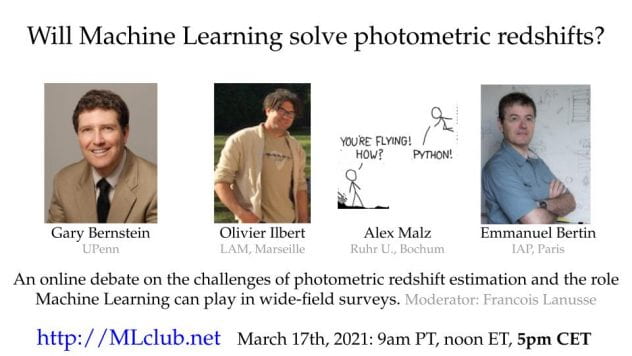
When: 9am PT, noon ET, 5pm CET
How: http://MLclub.net
Who:
- Gary Bernstein (UPenn)
- Olivier Ilbert (LAM, Marsielle)
- Alex Malz (Ruhr U., Bochum)
- Emmanuel Bertin (LAP, Paris)
Title: “Will Machine Learning solve photometric redshifts?”
Abstract: An online debate on the challenges of photometric redshift estimation and the role Machine Learning can play in wide-field surveys.
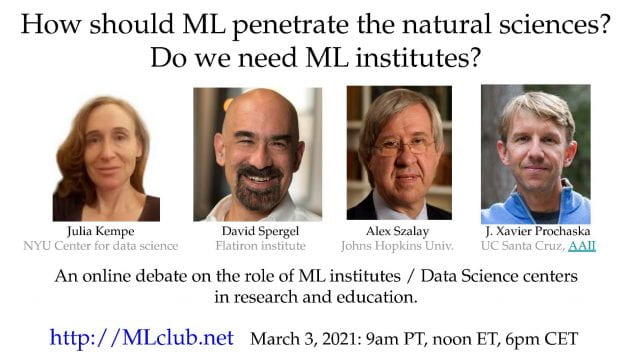
When: 9am PT, noon ET, 6pm CET
How: http://MLclub.net
Who:
- Julia Kempe (NYU Center for data science)
- David Spergel (Flatiron institute)
- Alex Szalay (John Hopkins Univ.)
- J. Xavier Prochaska (UC Santa Cruz, AAII)
Title: “How should ML penetrate the natural sciences? Do we need ML institutes?”
Abstract: An online debate on the role of ML institutes / Data Science centers in research and education.
Save the dates:
March 23, 9am – 12pm.
March 26, 9am – 12pm.
Check back with us. Details forthcoming!
Who:
- Francois Lanusse (CNRS; CEA Saclay)
- Marc Huertas-Company (U. Paris; IAC)
- Brice Menard (Johns Hopkins)
- J. Xavier Prochaska (UC Santa Cruz, AAII)
Title: “What is Deep Learning Teaching Astronomy?”
Abstract: The esteemed organizers of ML Club for Astronomy will discuss their perspectives on the positive and negative impacts of deep learning algorithms in Astronomy. Questions from the audience will be entertained.
Who: Sebastian Wetzel (Perimeter Institute for Theoretical Physics, Canada)
Title: “Siamese Neural Networks Learn Symmetry Invariants and Conserved Quantities”
Abstract: In this talk, we discuss Siamese Neural Networks (SNN) for similarity detection and apply them to examples in physics. These examples include special relativity, electromagnetism, and motion in a gravitational potential. The SNNs learn to identify data points belonging to the same events, field configurations, or trajectory of motion. In the process of learning which data points belong to the same event or field configuration, these SNNs also learn the relevant symmetry invariants and conserved quantities, which can be revealed by interpreting the latent space of the SNNs.
Who: Jared Kaplan (Johns Hopkins / OpenAI)
Title: “GPT-3, the most powerful language model, and neural scaling laws”
The work is based on this paper:
Who: François Charton (Facebook) and Amaury Hayat (Pasitech, Rutgers)
Title: “Learning maths from example with deep language models”
Abstract: In this presentation we show how to use deep language models to solve problems related to differential equations, namely solving ODE, and deriving properties of differential systems such as local stability or controllability. Our models have no built-in knowledge of mathematics and only learn through examples, yet they manage to achieve high performance on advanced problems.
The work is based on these 2 papers: